Linking Machine To Technician: A Journey To Improving Service Profitability
By Scott E. Day, principal consultant & business advisor, Transformational Strategies LLC
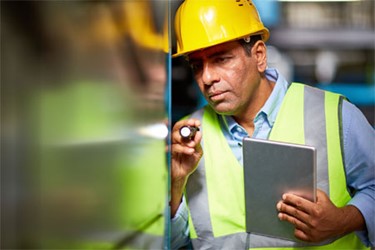
Maximizing service profitability comes from tightly linking the technician to the machine (equipment to be serviced) in a holistic way with artificial intelligence (AI) that is tightly integrated into the business. To maximize service profits, an Internet of Things (IoT) platform needs to be well thought out to maximize technician efficiency on every visit through a predictive maintenance program. The IoT platform is a piece that cannot stand on its own and must be tightly integrated with a highly disciplined supply chain strategy. Simply put, the technician needs to have the right part at the right time to preserve the life of the equipment and prevent unplanned equipment shutdowns. It’s a long journey but the good news is that the journey started two decades ago and is on a constantly accelerating pace as IoT and AI become more pervasive in field service applications.
A first step change at improving service profits came from Geo-based tools that improved route density. Improved route density reduces travel time between stops and some companies go one step further by using route guidance to ensure the technician doesn’t sit in traffic too long. However, decreasing travel time through geo-based algorithms only works when a technician’s day is scheduled with no disruptions. If technicians are performing both preventative maintenance and break-fix service, the travel time gains go out the window. An equipment shutdown generally takes precedent over routine maintenance from a customer service standpoint which typically puts preventative maintenance on the back burner. We all know that missed preventative maintenance causes further deterioration of the equipment and higher callbacks which causes less preventative maintenances, etc, etc. As callbacks rise and equipment conditions continue to deteriorate, service profits continue to decline as the breakdowns are typically more destructive in nature to the machine. To reverse that trend, it’s necessary to make the technician as intelligent as possible on every visit.
In early 2000, as cellular technology in the U.S. became more robust, companies began connecting machines to central databases and online expert systems linked to technicians. The expert system could identify the piece of equipment, the faults and event history, and offer the route technician solutions in almost real time to fix the equipment shutdown right with a 90 percent+ degree of accuracy. The near-real-time aspect was a stepped improvement at improving first time fix rates which in-turn allowed the technician more time to perform preventative maintenance thereby extending equipment life.
As the IoT has evolved, the static information from online expert systems of the past evolved with the addition of historical algorithms and trend analytics that became the basis of artificial intelligence. Artificial intelligence is developed using cloud computing to look at massive amounts of data and expose reproducible outcomes to the technician to improve equipment life. Enhancing that data with additional sensors and third-party data (i.e. temperature, humidity, etc.) can also give each a machine a unique signature to improve the relevance of the work to be done on each visit. Armed with this artificial intelligence, the technician now statistically has a better chance of fixing a shutdown the first time and also taking a more prescriptive approach to equipment maintenance over traditional check lists.
All of this assumes that the technician has the right part to make all the above happen. Stocking the correct parts at the right time without carrying inflated inventories on a service van is highly complex. Detailed part specifications add a new dimension to the condition-based intelligence that is at the core of the IoT platform and requires knowledge of the life expectancy of the parts. To make predictive maintenance as efficient as possible, the supply chain strategy must be mature with tightly controlled part specs and disciplined rules for changing suppliers in a closed loop fashion with the IoT platform. In other words, if the supplier changes and the spec for the new supplier’s part yields a different lifecycle than the previously part, the predictive maintenance algorithms must be updated to reflect this change as the new supplier’s part is integrated into use.
While all of this seems deep in technology, General Electric has been doing this for almost two decades with jet engines. If we step back up to the 10,000-foot level, the goal in maximizing service profitability is ensuring the technician is as efficient as possible on every single visit. Linking the machine to the technician in a holistic way and providing the technician with the artificial intelligence that comes from a well-structured IoT platform will maximize technician efficiency on every visit and maximize service profitability.